Data-Parallelism in Rust with the Rayon Crate
The Rayon crate is one of the most popular libraries for data-parallelism in Rust , providing a simple and efficient way to execute…
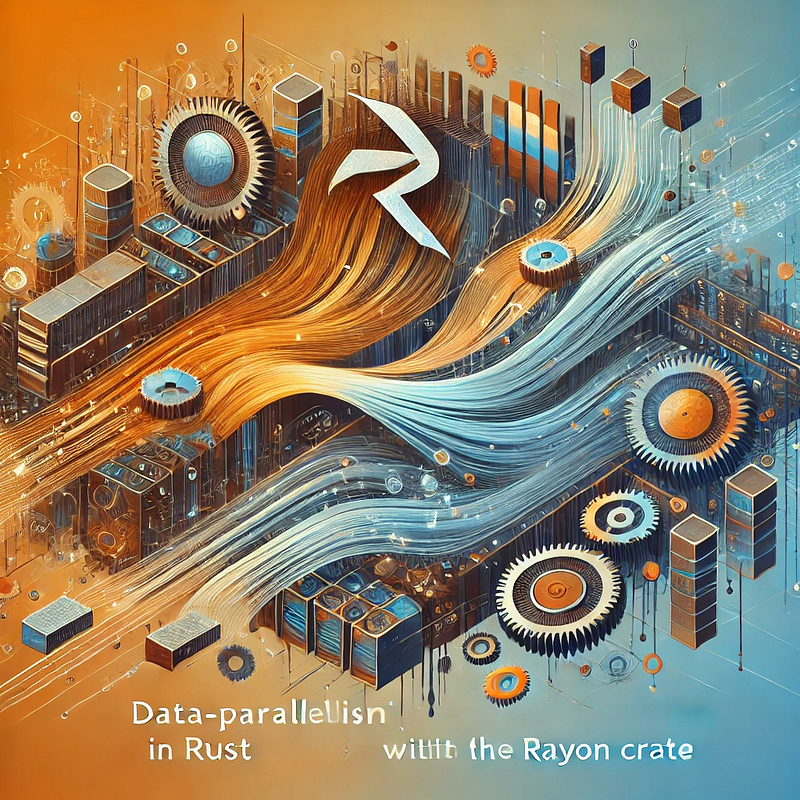
The Rayon crate is one of the most popular libraries for data-parallelism in Rust , providing a simple and efficient way to execute parallel computations.
In this article, we will explore its features with working examples.
Let´s go! 🦀
What is Data-Parallelism?
Data-parallelism involves distributing data across multiple processors to perform the same operation simultaneously. This contrasts with task parallelism, where different tasks are executed concurrently. Data-parallelism is particularly effective for operations on large datasets, where the same computation can be applied independently to different parts of the data.
Rayon Crate
Rayon is a data-parallelism library for Rust that makes it easy to convert sequential computations into parallel ones. It abstracts the complexity of managing threads, allowing developers to focus on their algorithms. Rayon works by dividing the data into chunks and processing them in parallel across multiple threads.
Key Features of Rayon
- Ease of Use: Provides parallel iterators that mirror Rust’s standard iterators.
- Automatic Chunking: Automatically divides data into chunks for parallel processing.
- Load Balancing: Distributes work evenly across threads to maximize efficiency.
- Safety: Ensures safe parallel execution without data races, leveraging Rust’s ownership and type system.
Getting Started with Rayon
First, add the Rayon crate to your Cargo.toml
:
[dependencies]
rayon = "1.5"
Next, import the Rayon prelude in your Rust file:
use rayon::prelude::*;
Examples
Example 1: Parallel Sum of an Array
Let's start with a simple example of calculating the sum of an array in parallel.
use rayon::prelude::*;
fn parallel_sum(arr: &[i32]) -> i32 {
arr.par_iter().sum()
}
fn main() {
let data = vec![1, 2, 3, 4, 5, 6, 7, 8, 9, 10];
let sum = parallel_sum(&data);
println!("The sum of the array is: {}", sum);
}
In this example, par_iter()
is used to create a parallel iterator. The sum()
method then performs the summation in parallel.
Example 2: Parallel Map and Filter
Suppose we want to double each element in an array and then filter out the even numbers. Here's how to do it with Rayon
use rayon::prelude::*;
fn parallel_map_filter(arr: &[i32]) -> Vec<i32> {
arr.par_iter()
.map(|&x| x * 2)
.filter(|&x| x % 2 == 0)
.collect()
}
fn main() {
let data = vec![1, 2, 3, 4, 5, 6, 7, 8, 9, 10];
let result = parallel_map_filter(&data);
println!("The filtered array is: {:?}", result);
}
Here, par_iter()
creates a parallel iterator, map()
doubles each element, and filter()
retains only even numbers. The collect()
method gathers the results into a Vec<i32>
.
Example 3: Parallel Sorting
Rayon also provides a way to sort data in parallel. Here's an example:
use rayon::prelude::*;
fn parallel_sort(arr: &mut [i32]) {
arr.par_sort();
}
fn main() {
let mut data = vec![10, 5, 8, 1, 7, 6, 3, 2, 4, 9];
parallel_sort(&mut data);
println!("The sorted array is: {:?}", data);
}
The par_sort()
method sorts the array in parallel, leveraging multiple threads to speed up the sorting process.
Advanced Usage
Custom Parallel Iterators
Rayon allows creating custom parallel iterators. Suppose you want to create a custom iterator that processes elements in pairs:
use rayon::prelude::*;
use rayon::iter::plumbing::*;
use std::sync::Arc;
struct PairChunks<'a, T: 'a> {
slice: &'a [T],
}
impl<'a, T> PairChunks<'a, T> {
fn new(slice: &'a [T]) -> Self {
PairChunks { slice }
}
}
impl<'a, T: Sync + 'a> ParallelIterator for PairChunks<'a, T> {
type Item = (&'a T, &'a T);
fn drive_unindexed<C>(self, consumer: C) -> C::Result
where
C: UnindexedConsumer<Self::Item>,
{
bridge_unindexed(self, consumer)
}
}
impl<'a, T: Sync + 'a> UnindexedProducer for PairChunks<'a, T> {
type Item = (&'a T, &'a T);
fn split(self) -> (Self, Option<Self>) {
if self.slice.len() < 2 {
(self, None)
} else {
let mid = self.slice.len() / 2;
(
PairChunks::new(&self.slice[..mid]),
Some(PairChunks::new(&self.slice[mid..])),
)
}
}
fn fold_with<F>(self, mut folder: F) -> F
where
F: Folder<Self::Item>,
{
for chunk in self.slice.chunks(2) {
if let [a, b] = chunk {
folder = folder.consume((a, b));
}
}
folder
}
}
fn main() {
let data = vec![1, 2, 3, 4, 5, 6, 7, 8, 9, 10];
let result: Vec<(&i32, &i32)> = PairChunks::new(&data).collect();
for (a, b) in result {
println!("Pair: ({}, {})", a, b);
}
}
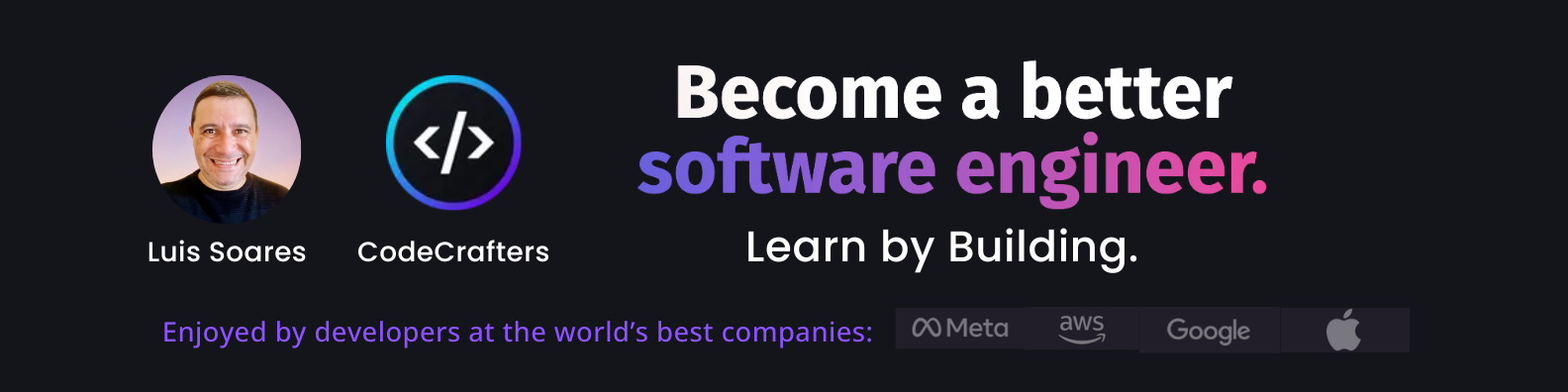
This example demonstrates creating a custom parallel iterator that processes elements in pairs. This flexibility allows for highly specialized parallel processing patterns.
Detailed Mechanics of Work-Stealing Scheduler
The work-stealing scheduler in Rayon is designed to maximize CPU utilization by dynamically balancing the workload among threads. Here’s a breakdown of how it functions:
- Task Decomposition and Splitting:
- When a parallel operation starts, Rayon decomposes the workload into smaller tasks. For instance, an array of 1,000 elements might be split into chunks of 100 elements each.
- This decomposition uses a divide-and-conquer approach, which recursively splits tasks until they reach a granularity that is efficient for parallel execution.
2. Double-Ended Queue (Deque):
- Each worker thread in Rayon has its own deque to store tasks.
- The deque supports push and pop operations from both ends, allowing flexible task management.
- When a thread finishes its tasks, it attempts to steal tasks from the back of another thread’s deque, ensuring minimal idle time and balancing the load across threads.
3. Work-Stealing Process:
- A worker thread, upon finding its deque empty, selects another thread at random and attempts to steal a chunk of tasks from the back of that thread’s deque.
- This approach minimizes contention and ensures that threads are not idling while there is work available.
Adaptive Chunking and Load Balancing
Rayon’s adaptive chunking mechanism is pivotal for achieving optimal performance:
- Dynamic Chunk Sizes:
- Instead of fixed-size chunks, Rayon dynamically adjusts chunk sizes based on the workload and system characteristics.
- Larger chunks are processed initially, and as the task progresses, the chunk sizes decrease, allowing finer granularity of work distribution.
- Balanced Work Distribution:
- By adjusting chunk sizes dynamically, Rayon ensures that the workload is evenly distributed across all threads.
- This approach prevents scenarios where some threads finish early and remain idle while others are still working on large chunks.
Example: Matrix Multiplication with Rayon
Matrix multiplication is a computationally intensive task that can benefit significantly from parallel processing. Here’s an example of how to implement parallel matrix multiplication using Rayon:
use rayon::prelude::*;
use std::time::Instant;
fn parallel_matrix_multiply(a: &[Vec<i32>], b: &[Vec<i32>]) -> Vec<Vec<i32>> {
let n = a.len();
let m = b[0].len();
let p = b.len();
assert_eq!(a[0].len(), p);
let mut result = vec![vec![0; m]; n];
result.par_iter_mut().enumerate().for_each(|(i, row)| {
for j in 0..m {
row[j] = (0..p).map(|k| a[i][k] * b[k][j]).sum();
}
});
result
}
fn main() {
let n = 500;
let m = 500;
let p = 500;
let a: Vec<Vec<i32>> = (0..n).map(|_| (0..p).map(|_| rand::random::<i32>() % 10).collect()).collect();
let b: Vec<Vec<i32>> = (0..p).map(|_| (0..m).map(|_| rand::random::<i32>() % 10).collect()).collect();
let start = Instant::now();
let result = parallel_matrix_multiply(&a, &b);
let duration = start.elapsed();
println!("Time taken: {:?}", duration);
println!("Result (first row): {:?}", result[0]);
}
In this example:
- Data Initialization: We initialize two matrices
a
andb
with random integers. - Parallel Multiplication: The
par_iter_mut
method is used to iterate over rows of the result matrix in parallel. Each row computation involves an inner loop over columns, where the dot product of the row and column is calculated. - Performance Measurement: The
Instant
module measures the time taken for the multiplication.
Example: Parallel Merge Sort with Rayon
Merge sort is a classic sorting algorithm that can be efficiently parallelized. Here’s how to implement parallel merge sort with Rayon:
use rayon::prelude::*;
use std::time::Instant;
fn parallel_merge_sort<T: Ord + Send>(mut arr: Vec<T>) -> Vec<T> {
let len = arr.len();
if len <= 1 {
return arr;
}
let mid = len / 2;
let (left, right) = arr.split_at_mut(mid);
rayon::join(|| parallel_merge_sort(left.to_vec()), || parallel_merge_sort(right.to_vec()))
.into_iter()
.flatten()
.collect()
}
fn merge<T: Ord>(left: Vec<T>, right: Vec<T>) -> Vec<T> {
let mut merged = Vec::with_capacity(left.len() + right.len());
let mut left_iter = left.into_iter();
let mut right_iter = right.into_iter();
let mut left_next = left_iter.next();
let mut right_next = right_iter.next();
loop {
match (left_next, right_next) {
(Some(l), Some(r)) => {
if l <= r {
merged.push(l);
left_next = left_iter.next();
} else {
merged.push(r);
right_next = right_iter.next();
}
}
(Some(l), None) => {
merged.push(l);
merged.extend(left_iter);
break;
}
(None, Some(r)) => {
merged.push(r);
merged.extend(right_iter);
break;
}
(None, None) => break,
}
}
merged
}
fn main() {
let data: Vec<i32> = (0..1_000_000).map(|_| rand::random::<i32>() % 100).collect();
let start = Instant::now();
let sorted_data = parallel_merge_sort(data);
let duration = start.elapsed();
println!("Time taken: {:?}", duration);
println!("First 10 elements: {:?}", &sorted_data[..10]);
}
In this example:
- Data Initialization: A large vector of random integers is created.
- Parallel Merge Sort:
- The
parallel_merge_sort
function recursively splits the array into smaller sub-arrays. - The
rayon::join
function concurrently sorts the left and right halves. - The
merge
function combines the sorted halves into a single sorted array.
2. Performance Measurement: The time taken for the sorting operation is measured and printed.
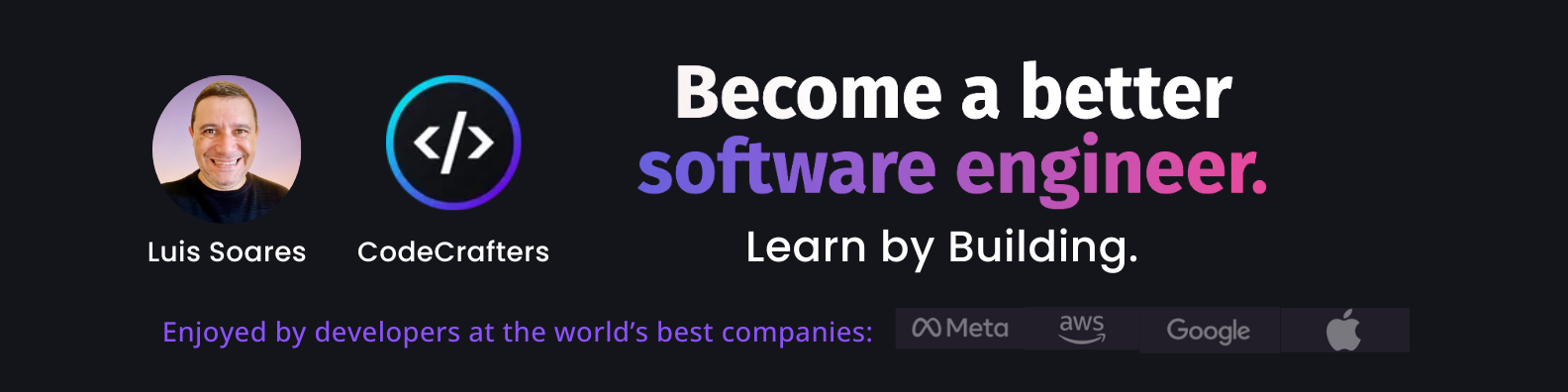
🦀 Ready to go beyond tutorials?
CodeCrafters is where software engineers become exceptional.
🔑 Sharpen Your Fundamentals
Recreate legendary software like Git and databases. Build the foundations that separate good developers from great ones.
⚡ Challenge Yourself
Work on projects that push your limits and build the confidence to tackle anything.
🌍 Learn from the Best
Join a community of engineers from Meta, Google, AWS, and more, sharing insights and solutions to real problems.
🚀 Future-Proof Your Career
Strengthen your skills through deliberate practice and become the developer everyone wants on their team.
This is your fast track to becoming a confident, standout engineer.
Stop practicing. Start mastering.
Join CodeCrafters Today!
🚀 Discover More Free Software Engineering Content! 🌟
If you enjoyed this post, be sure to explore my new software engineering blog, packed with 200+ in-depth articles, 🎥 explainer videos, 🎙️ a weekly software engineering podcast, 📚 books, 💻 hands-on tutorials with GitHub code, including:
🌟 Developing a Fully Functional API Gateway in Rust — Discover how to set up a robust and scalable gateway that stands as the frontline for your microservices.
🌟 Implementing a Network Traffic Analyzer — Ever wondered about the data packets zooming through your network? Unravel their mysteries with this deep dive into network analysis.
🌟Implementing a Blockchain in Rust — a step-by-step breakdown of implementing a basic blockchain in Rust, from the initial setup of the block structure, including unique identifiers and cryptographic hashes, to block creation, mining, and validation, laying the groundwork.
And much more!
✅ 200+ In-depth software engineering articles
🎥 Explainer Videos — Explore Videos
🎙️ A brand-new weekly Podcast on all things software engineering — Listen to the Podcast
📚 Access to my books — Check out the Books
💻 Hands-on Tutorials with GitHub code
🚀 Mentoship Program
👉 Visit, explore, and subscribe for free to stay updated on all the latest: Home Page
LinkedIn Newsletter: Stay ahead in the fast-evolving tech landscape with regular updates and insights on Rust, Software Development, and emerging technologies by subscribing to my newsletter on LinkedIn. Subscribe Here
🔗 Connect with Me:
- LinkedIn: Join my professional network for more insightful discussions and updates. Connect on LinkedIn
- X: Follow me on Twitter for quick updates and thoughts on Rust programming. Follow on Twitter
Wanna talk? Leave a comment or drop me a message!
All the best,
Luis Soares
luis@luissoares.dev
Lead Software Engineer | Blockchain & ZKP Protocol Engineer | 🦀 Rust | Web3 | Solidity | Golang | Cryptography | Author